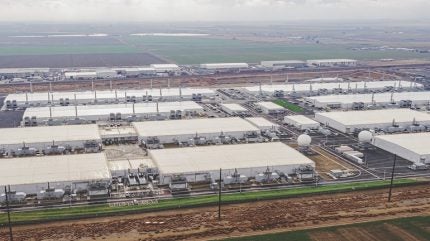
Debates about the impact of artificial intelligence on the energy transition are commonplace and show little sign of letting up, at least in the short term.
They also centre on one major conundrum, a circle that needs squaring. The “AI Age” will accelerate the shift to net zero by driving improvements in energy production, transmission, and usage, but at the same time it may slow this progress due to the high energy demands of data centres and networks supporting AI systems. How can the sector unlock this potential, without making a rod for its own back?
AI: not the main driver, not just renewables, not new
It is important to note though that policy, rather than AI, primarily drives the pace of the energy transition. Moreover, AI is not a tool exclusive to green energy developers; it is also being used in oil and gas production to improve efficiency, safety, and sustainability. That said, while AI-based tools and algorithms are often transferable across renewable energy sites, their application is more limited in oil and gas due to the unique nature of geological formations.
Much is currently being made of AI and its place in the energy sector. We are now at a pivotal moment where the large-scale adoption of AI into operating environments is materialising. Significant barriers – such as resistance to change, as well as concerns over technical and cybersecurity issues – mean this will inevitably take time. Additionally, it’s challenging to distinguish the specific impact of AI investment from broader digitalisation efforts by energy companies.
Despite this, DNV is closely monitoring developments and is actively involved in AI innovation for the energy industry, leading initiatives to ensure the safety and reliability of AI-powered systems. As we detailed in our most recent Energy Transition Outlook, AI is subject to Amara’s Law: its impact is often overestimated in the short term but underestimated in the long run.
Much of today’s AI hype focuses on generative AI, particularly large language models (LLMs). Their direct use in energy is limited to low-risk applications like staff productivity tools and early experimental projects, although the risks increase as the outcomes desired by the associated use cases become safety-critical.
AI that is relevant to energy systems generally falls under “discriminatory AI” – which includes applications like computer vision, forecasting, predictive maintenance – and design optimisation, which is collectively referred to
as “industrial AI”. These systems are already in use, and as AI methods improve, generative AI is expected to be increasingly applied to industrial settings.
The energy footprint of AI
It is widely assumed that the hardware used to train AI models – particularly graphics processing units (GPUs) – consumes significant amounts of energy. Training GPT-3, a precursor to ChatGPT, required around 1300 MWh of electricity, equivalent to the monthly energy use of 1450 average US households. On the surface this seems substantial, but it is only a small fraction of the total energy consumption of companies like Microsoft, whose energy use in 2022 reached 18.6 TWh.
In the coming years the training and use of AI is expected to grow dramatically, and it could exceed $1 trillion in market value by 2030, roughly five to six times its current size. However, energy consumption is unlikely to rise at the same rate, as there are ongoing efforts to develop “responsible AI” that focuses on efficiencies. Beyond hardware efficiencies, advancements in machine learning architectures, better algorithms, and cloud computing – particularly in regions with cleaner, more efficient energy – are expected to further reduce AI’s energy demands.
Although research into AI’s energy growth versus efficiency improvements is still developing, current data centre energy usage offers insights. According to the International Energy Agency, in 2022, data centres, cryptocurrencies, and AI consumed approximately 460 TWh of electricity, roughly 2% of global electricity demand. By 2026, this figure is expected to rise to 800 TWh, or 2.4% of global demand. In our energy model, AI-related data centre usage falls under commercial appliances and lighting, representing about 7.5% of this category. Within that figure, regional differences in demand are inevitable, with higher growth in Europe, North America, and China, where AI adoption is accelerating.
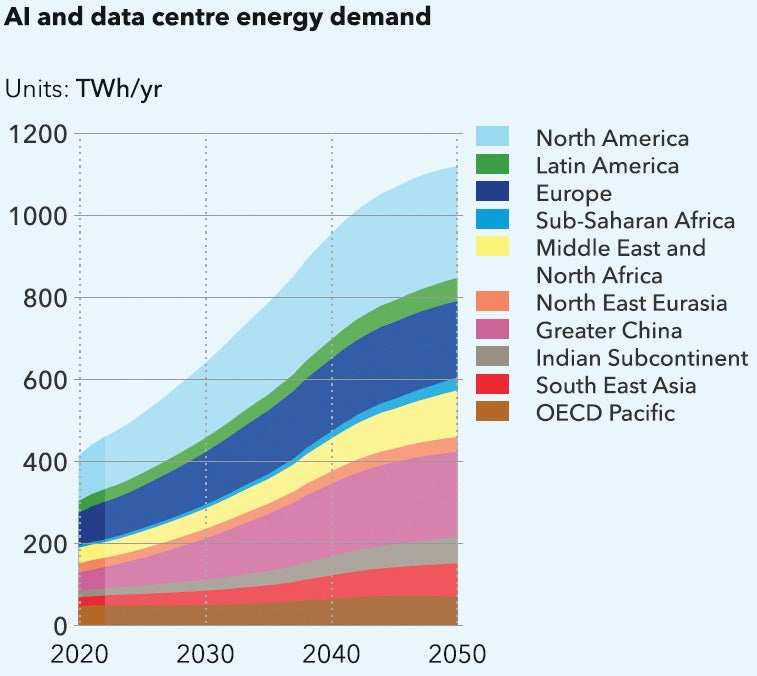
In the broader context, this remains a relatively small portion of global electricity demand. By 2050, AI is forecast to account for just under 5% of electricity demand in the buildings sector and nearly 2% of overall global electricity usage. However, global electricity demand is expected to more than double by mid-century, meaning AI’s energy consumption could triple during this period, despite ongoing efficiency gains. These forecasts carry significant uncertainties, which will be ironed out with further research and actual evidence.
Current discussions primarily focus on the direct energy consumption of AI and the potential efficiency gains at data centres and in AI hardware. However, a larger and more meaningful conversation lies in comparing AI’s energy and emissions footprint with the many efficiencies it is expected to bring to daily life, including energy use.
A revolution that’s well underway
Even at this early stage, AI technology is driving significant advancements in areas such as power grid management, materials science, renewable energy siting, and environmental impact assessments.
It has the potential to revolutionise transmission and distribution system operations and assist with grid planning, line routing, transformer placement, asset management, and day-to-day operations. AI models help generate synthetic data for grid planning, visualise grid designs, predict maintenance needs, and analyse sensor data to identify potential issues early. Power companies like PG&E and Duke Energy are already using AI for infrastructure inspections and anomaly detection, enhancing grid reliability and reducing downtime.
AI also improves real-time assessments of power transmission line capacity through dynamic line rating, which uses environmental and load data to provide more accurate – and often higher – capacity ratings. AI-based models of customer load profiles are proving useful in grid modeling, demand response, and overall grid operations. Competitions like Learning to Run a Power Network (L2RPN) highlight AI’s potential to optimise complex grid operations, hinting at future automation.
AI plays a crucial role in energy storage by optimising battery charge and discharge cycles, reducing energy losses, and extending the lifespan of battery systems. DNV’s Battery AI service predicts battery life based on usage patterns, while AI-driven simulations help determine the ideal size and placement of energy storage systems. Machine learning is also accelerating the discovery of novel battery chemistries, further advancing storage technology.
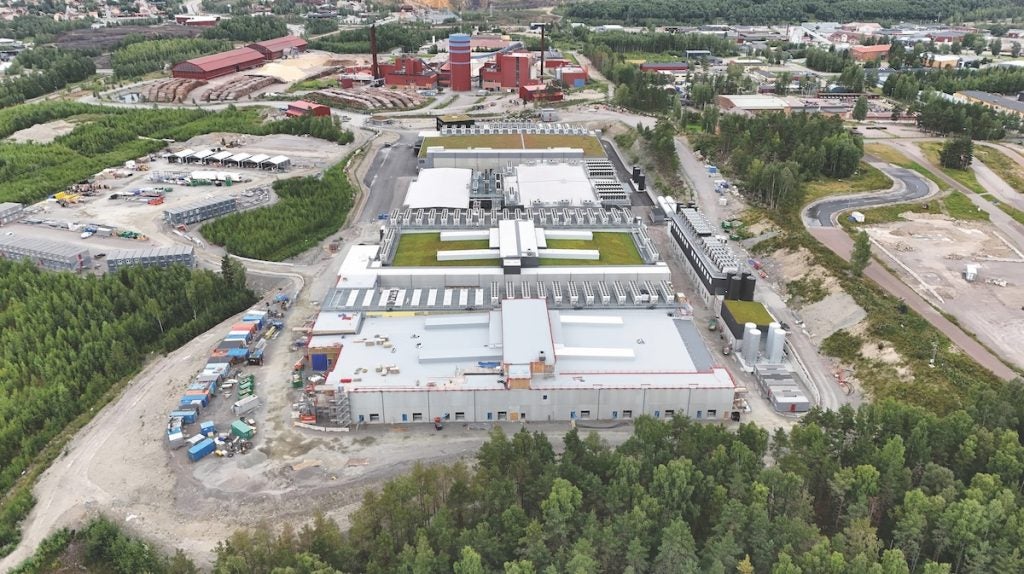
Machine learning is key to optimising the balance between energy supply and demand, improving the financial viability of renewable energy, and integrating it into the grid. Companies like DNV’s Solcast provide solar irradiance data and forecasts using AI algorithms, while DNV’s Forecaster service employs advanced statistical methods and machine learning to deliver short-term forecasting for wind and solar sites worldwide.
AI applications in optimising the siting and design of renewable energy installations are widespread. For example, researchers in China have developed a machine learning model that uses satellite and sunshine data to optimise the placement of double-sided solar panels. In the USA, DNV partner HST employs AI-based decision engines and data analytics to optimise large-scale solar installations, connecting developers with energy buyers and other key project stakeholders.
AI is transforming materials science, particularly in such areas as discovering and selecting materials for power generation components and new battery chemistries. The US National Renewable Energy Laboratory (NREL), for instance, has used invertible neural networks to significantly accelerate the design process for wind turbine blades. Additionally, AI is being used to assess molecules for their stability and chemical properties to enhance carbon capture technologies and identify new approaches for carbon capture and storage (CCS) projects.
AI can also streamline complex and time-consuming approval and permitting processes. For example, in the nuclear sector, AI could enhance reactor design, plant operations, and training. AI is also being applied in environmental planning, where it utilises sensor networks and drones for biodiversity monitoring, aiding data visualisation and report generation. This could significantly speed up environmental assessments and permitting processes.
A double-edged sword
While AI promises to drive substantial advancements in the energy sector – for example, enhancing power grid management, optimising energy storage, accelerating materials discovery, and improving renewable energy siting – it also brings challenges.
Leaving the moral and ethical debate to one side, the technology’s own growing energy footprint must be monitored and accounted for, or else it could become a blocker rather than an enabler.
The key question remains: how can we balance AI’s transformative potential in the energy transition with its rising energy demands?
Though AI is not the primary driver of the transition to net zero, its role is significant, and ongoing efforts to improve energy efficiency, particularly in data centres and hardware, are essential. Its long-term impact on both the energy sector, and broader society, will likely be underestimated, and its influence will continue to grow in tandem with advancements in responsible AI development.